Breast Cancer Detection using Image Segmentation

DOI:
https://doi.org/10.54060/pcc.2023.1Keywords:
Deep Learning, Image Segmentation, Sematic Segmentation, Transfer Learning, Case ReportAbstract
Breast cancer is the most prevalent kind of malignancy in women, and diagnostic systems that apply artificial intelligence algorithms for breast imaging have shown positive results. Two methods that increase the precision of detecting breast tu-mors from mammography images are a multiclass support vector machine model and a deep convolutional neural network (DCNN) using K-means clustering. To accurately diagnose breast tumors, the pectoral muscle (PM) border must still be dis-tinguished from the rest of the breast tissue. By merging the transfer learning model with a number of pre-trained CNN structures, this research offers an Ensemble-Net model for distinguishing the PM boundary from the rest breast region in mammograms. The segmentation procedure consists of 2 steps. According to the input, various regions of interest are formed in the initial phase and include the object.
Downloads
References
Szegedy C, Ioffe S, Vanhoucke V, Alemi A. Inception-v4, Inception-ResNet and the impact of residual connections on learning. Proc Conf AAAI Artif Intell [Internet]. 2017;31(1). Available from: http://dx.doi.org/10.1609/aaai.v31i1.11231.
Simonyan K, Zisserman A. Very deep convolutional networks for large-scale image recognition [Internet]. arXiv [cs.CV]. 2014. Available from: http://arxiv.org/abs/1409.1556.
Krizhevsky A, Sutskever I, Hinton GE. ImageNet classification with deep convolutional neural networks. Commun ACM [Internet]. 2017;60(6):84–90. Available from: http://dx.doi.org/10.1145/3065386.
LeCun Y, Haffner P, Bottou L, Bengio Y. Object recognition with gradient-based learning. In: Shape, Contour and Grouping in Computer Vision. Berlin, Heidelberg: Springer Berlin Heidelberg; 1999. p. 319–45.
Rajaraman S, Zamzmi G, Antani SK. Novel loss functions for ensemble-based medical image classification. PLoS One [In-ternet]. 2021;16(12): e0261307. Available from: http://dx.doi.org/10.1371/journal.pone.0261307.
Scohy A, Anantharajah A, Bodéus M, Kabamba-Mukadi B, Verroken A, Rodriguez-Villalobos H. Low performance of rapid antigen detection test as frontline testing for COVID-19 diagnosis. J Clin Virol [Internet]. 2020;129(104455):104455. Avail-able from: http://dx.doi.org/10.1016/j.jcv.2020.104455.
Jayamohan H, Lambert CJ, Sant HJ, Jafek A, Patel D, Feng H, et al. SARS-CoV-2 pandemic: a review of molecular diagnostic tools including sample collection and commercial response with associated advantages and limitations. Anal Bioanal Chem [Internet]. 2021;413(1):49–71. Available from: http://dx.doi.org/10.1007/s00216-020-02958-1.
Wang G, Liu X, Shen J, Wang C, Li Z, Ye L, et al. Author Correction: A deep-learning pipeline for the diagnosis and dis-crimination of viral, non-viral and COVID-19 pneumonia from chest X-ray images. Nat Biomed Eng [Internet]. 2021;5(8):943. Available from: http://dx.doi.org/10.1038/s41551-021-00787-w.
Wang X, Peng Y, Lu L, Lu Z, Bagheri M, Summers RM. ChestX-Ray8: Hospital-scale chest X-ray database and benchmarks on weakly-supervised classification and localization of common thorax diseases. In: 2017 IEEE Conference on Computer Vision and Pattern Recognition (CVPR). IEEE; 2017.
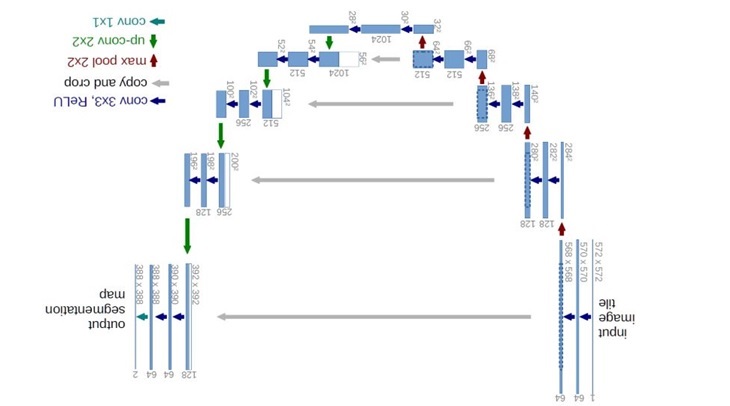
Downloads
Published
How to Cite
CITATION COUNT
Issue
Section
License
Copyright (c) 2023 Mohit Sethi, Dr. P. Singh

This work is licensed under a Creative Commons Attribution 4.0 International License.